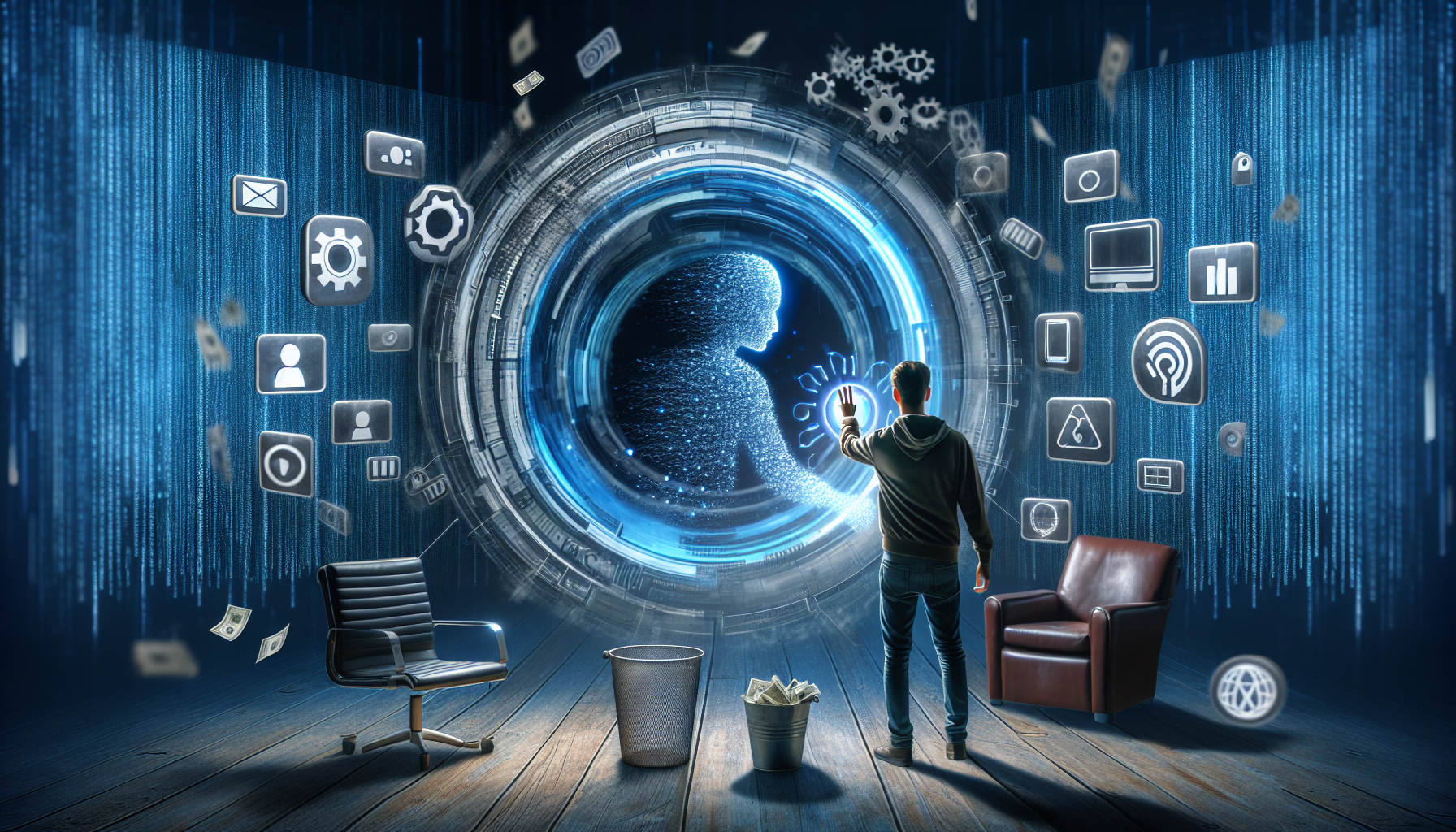
Artificial intelligence (AI) is reshaping the landscape of how we interact, work, and secure our digital lives. However, along with the immense benefits, there are significant dangers of AI that we cannot afford to overlook. The concerns surrounding AI security and the safety of our personal data are growing, highlighting the need for awareness and proactive measures to guard against potential threats. This vital topic not only interests us but demands our attention, as the implications of ignoring the dangers of AI could be far-reaching and detrimental to our privacy and cyber security.
As we delve into the nuances of this topic, we will explore the importance of understanding AI and data privacy, including how AI uses personal data and the inherent privacy concerns and risks associated with it. Our journey will also lead us through the best practices for protecting our data, the importance of regulatory compliance, and the need for responsible AI development. By providing a roadmap of these critical areas, our aim is to equip readers with the knowledge to navigate the complexities of artificial intelligence security and to foster a safer digital environment for all.
Understanding AI and Data Privacy
As we explore the realm of AI and data privacy, it’s crucial to recognise the intricate balance between leveraging artificial intelligence and safeguarding personal data. AI systems, by their nature, can intensify existing security risks, making them more challenging to manage. This complexity arises from the technological sophistication of AI, which differs significantly from traditional IT systems we might be familiar with. For instance, AI often relies on extensive third-party code and supplier relationships, adding layers of dependency and potential vulnerability.
From a human perspective, the diversity in the backgrounds of those developing and deploying AI—from software engineers to data scientists and domain experts—means that there’s a broad spectrum of security practices and data protection awareness. This diversity can lead to inconsistencies in understanding and implementing robust security measures.
Moreover, AI’s capability to process vast amounts of personal data heightens the risk of inadvertent exposure. Techniques like model inversion and membership inference attacks can reveal personal data from training datasets, underscoring the need for stringent security protocols.
In response to these challenges, adhering to the data minimisation principle is essential. This principle mandates processing only the necessary amount of personal data, thereby reducing potential risks. Employing techniques such as feature selection, perturbation, synthetic data, and federated learning can help in aligning with this principle while maintaining the efficacy of AI systems.
Understanding and mitigating the privacy risks associated with AI is not just about technical measures; it’s about fostering a culture of privacy that respects and protects individual data rights. This approach will not only comply with regulatory requirements but also build trust in AI technologies, ensuring their responsible and ethical use in society.
How AI Uses Personal Data
Artificial intelligence (AI) thrives on extensive datasets to improve its algorithms. In our endeavour to understand how AI uses personal data, we must consider various data collection methods and the necessity for large data sets.
Data Collection Methods
AI data collection involves gathering, organising, and curating data from diverse sources to train algorithms. This process can range from using open source datasets like those available on Kaggle or Data.Gov, which provide quick access to large volumes of data, to employing synthetic datasets that mimic real-world data without the associated privacy risks. Another effective method is transfer learning, where a pre-existing algorithm serves as the foundation for training a new algorithm, significantly saving time and resources. For more specific needs, collecting raw data directly from the field might be essential, ensuring the data is perfectly tailored to the AI’s requirements.
The Need for Large Data Sets
The development of machine learning algorithms heavily relies on large, diverse datasets. These datasets allow the algorithms to draw numerous entities, relationships, and clusters, enriching the correlations made during the learning process. From image and video datasets that enhance pattern recognition to complex 3D point cloud data for autonomous vehicles, the variety and volume of data directly influence the effectiveness and reliability of AI systems. Ensuring the quality and relevance of these datasets is crucial, as they form the backbone of AI’s learning and operational capabilities.
By integrating these methods and acknowledging the need for comprehensive data sets, we can better equip AI systems to handle tasks that were once thought to be exclusive to human intelligence, thereby enhancing both performance and security.
Privacy Concerns and Risks Associated with AI
Data Breaches and Unauthorised Access
We often hear about the potential for data breaches and unauthorised access when it comes to AI. These platforms, which store vast amounts of sensitive data like personal and health records, are prime targets for cyber attacks. Internally, weak security protocols, inadequate encryption, and lack of proper access controls can leave AI systems vulnerable. Externally, attackers can exploit these weaknesses, leading to significant cyber security risks and potential GDPR infringements.
Surveillance and Monitoring
The use of AI in surveillance significantly raises data privacy and security concerns. Increasingly, governments and organisations employ AI-driven surveillance tools, intensifying fears about how personal data is utilised and scrutinised. This overreach can lead to invasive monitoring, where the line between public safety and personal privacy blurs, challenging ethical norms and potentially infringing on individual freedoms.
Bias and Discrimination Concerns
AI systems can inadvertently perpetuate existing societal biases, leading to discrimination in critical areas like employment and law enforcement. If the data used to train these systems is biased, the AI’s algorithms can further these prejudices, resulting in unfair outcomes. This is particularly concerning in technologies like facial recognition, which have been shown to suffer from accuracy issues, disproportionately affecting minority groups. Ensuring fairness in AI involves rigorous testing and the diversification of training datasets to mitigate these biases effectively.
Best Practices for Protecting Your Data
Implementing Privacy by Design
We must integrate privacy and data protection principles from the very beginning of the AI system’s lifecycle. By doing so, we not only enhance the security of personal data but also build trust with users who are more likely to adopt AI systems that prioritise their privacy. This approach involves embedding privacy into the design process, ensuring end-to-end security, and maintaining transparency about how personal data is used.
Anonymity and Aggregating Data
Anonymisation and aggregation are crucial for protecting privacy. Anonymisation makes it impossible to identify individuals from data sets, which is essential for maintaining privacy and reducing data protection risks. Aggregation, on the other hand, combines data to provide summary information without exposing individual details, thus protecting personal identities while still allowing for valuable insights.
Data Minimisation and Retention Policies
Adhering to data minimisation principles requires us to collect and retain only the data necessary for specific purposes. This not only complies with legal requirements but also reduces the potential for data breaches. Regular audits and the application of technologies like data analytics tools help in enforcing these principles effectively. Additionally, setting clear data retention policies ensures that data is not held longer than necessary, thereby minimising risks associated with data storage and processing.
Regulatory Compliance and Responsible AI Development
GDPR and Data Protection Laws
We are increasingly aware of the integral role that data protection laws, such as the General Data Protection Regulation (GDPR), play in the lifecycle of AI systems. These regulations ensure that personal data is processed lawfully, promoting transparency and safeguarding against misuse. By aligning AI practices with GDPR, we ensure that AI systems do not just operate efficiently but also ethically, respecting user privacy at every stage of development and deployment.
Building Ethical and Transparent AI Systems
To foster trust and accountability in AI, it’s crucial to develop systems that are not only technically proficient but also ethically sound. This involves implementing robust mechanisms for transparency and auditability, particularly in high-risk scenarios. Ensuring that AI systems are understandable and their actions justifiable is essential for maintaining public trust and adherence to ethical standards. By integrating these practices, we commit to responsible AI development that upholds the dignity and rights of all individuals involved.
Our Thoughts
Through this exploration of the intersection between artificial intelligence, data privacy, and security, we’ve underscored the complex balance required to harness the benefits of AI while safeguarding our digital lives against inherent risks. The discussion has traversed the landscape of AI’s use of personal data, highlighting the critical need for stringent data protection practices, regulatory compliance, and the cultivation of responsible AI development. By delving into the potential vulnerabilities and privacy concerns, along with best practices for data protection, this article aims to arm readers with the insights necessary to navigate the complexities of AI with greater awareness and preparedness.
In acknowledging the dual facets of AI as both a tool for advancement and a potential threat to privacy, the call for a proactive stance on ethical AI practices and data protection becomes ever more pressing. As we stand at the cusp of technological evolution, the collective responsibility to advocate for and implement measures that safeguard privacy and promote ethical use of AI is paramount. With the implications of AI and data usage poised to redefine societal norms, the discussion does not end here but rather marks a critical juncture for further inquiry, reflection, and action towards nurturing an environment where technology serves humanity’s best interests without compromising our digital security and privacy.